生成AIが実社会に大きな影響を与えています。数学的に見ると、生成AIは確率分布です。その内部ではニューラルネットによって文書や画像の生成確率が計算され、モデルごとに出力傾向に個性があります。私たちは、日々提案される生成AIモデルの類似性を把握するため、確率分布の空間における位置関係を反映するような座標を計算する統計的手法を提案しました。情報幾何学のアプローチにより、モデル間のKLダイバージェンス(確率分布間のずれをエントロピーで測った指標)を近似する「生成AIの地図」を比較的小さい計算コストで作成できます。
約1000個の言語モデルの地図
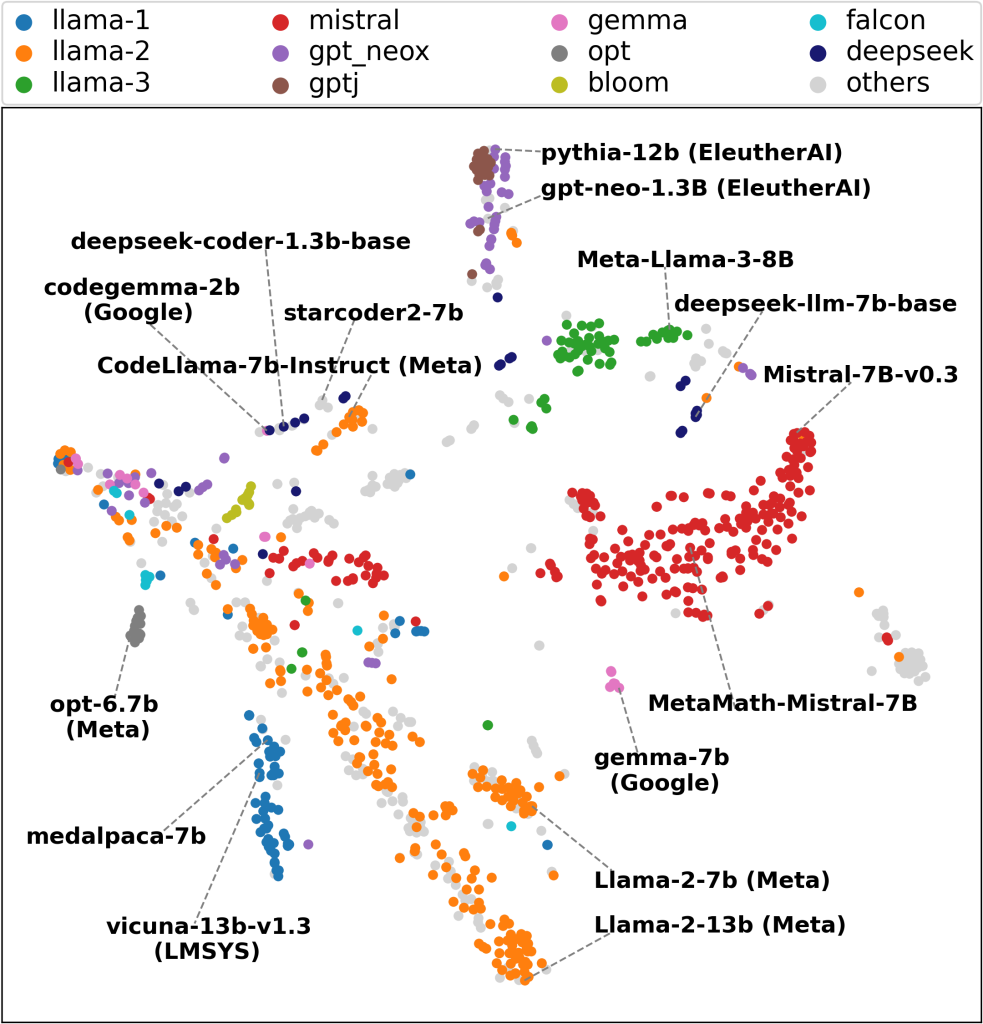
参考文献
- Momose Oyama, Hiroaki Yamagiwa, Yusuke Takase, Hidetoshi Shimodaira, Mapping 1,000+ Language Models via the Log-Likelihood Vector, https://arxiv.org/abs/2502.16173 (accepted to ACL2025 main)